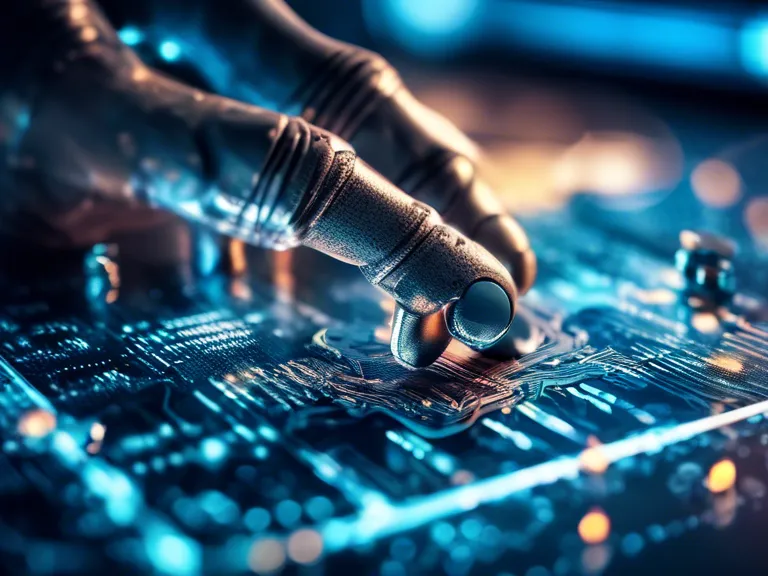
Machine learning applications have revolutionized various industries by enabling companies to leverage data in making informed decisions. In the financial sector, machine learning plays a crucial role in deposit risk management. By analyzing vast amounts of data, machine learning models can predict and manage deposit risks effectively, helping financial institutions minimize losses and optimize their operations.
Understanding Deposit Risk Management
Deposit risk management is a critical aspect of banking operations that involves assessing and mitigating the risks associated with deposits. Banks and financial institutions rely on customer deposits as a primary source of funding for their lending activities. However, deposit risks can arise from various factors, such as interest rate fluctuations, economic conditions, regulatory changes, and customer behavior.
Role of Machine Learning in Deposit Risk Management
Machine learning algorithms can analyze historical data to identify patterns and trends that traditional risk management methods may overlook. By using advanced analytics techniques, such as regression analysis, clustering, and neural networks, machine learning models can assess the likelihood of deposit defaults, detect fraudulent activities, and predict customer behavior.
Applications of Machine Learning in Deposit Risk Management
Credit Scoring: Machine learning models can analyze customer data to assess creditworthiness and assign credit scores, helping banks make informed decisions on loan approvals and interest rates.
Fraud Detection: Machine learning algorithms can detect fraudulent activities, such as identity theft and account takeover, by analyzing transaction data and customer behavior patterns.
Churn Prediction: By analyzing customer interactions and transaction history, machine learning models can predict the likelihood of customers closing their accounts or shifting funds to other institutions.
Portfolio Management: Machine learning can optimize portfolio management by analyzing market data, customer preferences, and risk profiles to allocate funds effectively and maximize returns.
Benefits of Using Machine Learning in Deposit Risk Management
Improved Accuracy: Machine learning models can analyze vast amounts of data quickly and accurately, providing more precise risk assessments compared to traditional methods.
Real-time Monitoring: Machine learning algorithms can continuously monitor deposit accounts and transactions, enabling banks to detect and respond to risks promptly.
Cost Efficiency: By automating risk management processes, machine learning can reduce operational costs and improve efficiency in managing deposit risks.
Enhanced Customer Experience: Machine learning models can personalize services based on customer preferences and behaviors, leading to a better overall customer experience.
Challenges and Considerations
While machine learning offers significant benefits in deposit risk management, there are challenges and considerations to address. These include data privacy and security concerns, regulatory compliance requirements, model interpretability, and the need for ongoing monitoring and validation of machine learning models.
In conclusion, machine learning applications in deposit risk management have the potential to transform how financial institutions assess and mitigate risks associated with customer deposits. By leveraging advanced analytics and predictive modeling techniques, banks can enhance their risk management practices, improve decision-making processes, and ultimately, optimize their financial performance.
american-boffin.com
bfbchamp.com
democraticcoma.com
tigrepelvar.com
charpoles.com
derbywheelblazers.com
fansfocus.net
guildnow.com
hediyeteyze.com
isprimecdn.com
kiira-korpi.net
manutd24.com
mediumtylerhenry.com
mishanghai.org
savethreestrikes.com
smilesbydavis.com
10puntos.net
band-shirt.com
icelandtrails.com
paulmarioday.com
thefunnynanny.com
bebovino.com
car-trackers.com
colbykeller.com
companyregistrationuk.com
dayoftears.com
entamee.com
kuttikattil.com
litjunkies.com
ljshen.com
nostalgic-radio.com
pcgameshackcenter.com
rachelboggia.com
vanessagritton.com
veraandrose.com
vernissage-zermatt.com
almagnon.com
angelicmedium.com
ascensoya.com
ascrenewables.com
bar-flat.com
beerandgluesticks.com
disorderedtimes.com
dokodepot.com
epochpr.com
essaylancers.com
gravure-movie.com
hawkeyespacover.com
hgwlab.com
itinerecoop.com
jeremyendoart.com
kananroadband.com
loguecorporate.com
nishikawaguchi-dosukebeworld.com
pikkupunapippuri.com
publishmyblog.com
rebius-kawai.com
salinggrove.com
sean-santiago.com
sitesbydawn.com
styledbyrida.com
thegolfcoastonline.com
thisismymilwaukee.com
breadboardmaniac.com
takasuapp.com
Anostomodo
Bonet Scissors
Cora Viral
Dippity Do Dog Mobile
Group Tie
Newberry County History
Oslo Velo
Planned and Present
Wilshire Language
CFL Magazine
Corner Kitchen
Glass of Win
Hydeout at the Wharf
Lotus Relocation
My Dee Dee's
Platinum Avtomaty Wulkan
Pride Outside
Rollout Reviews
Smile to Africa Adventure
ABC Trcker
Aloha Botts and Tots
America Noticias
Are You Kenilworthy
Ask My Condo
Charm Shiki
El Akhbaar
Health Cursor
Home Again Creative
Mi Punto PE
Patt and Billy
Rustique Online
SS Pro HK
Steamworks Espresso
Targeted Fat Loss Training
Team Hamano
Used Cars Buying
Dave Tries Ballet
Buon Grande
Criacao Sites
Perry Perkins Books
Writing Essay in AU
Ka Soku
Blood is Blood Movie
Eleanor Writes Things
The Happy Prince Beirut
Town of Witless Bay
Online Igrovoi Club
Trigeminal Neuralgia - Ronald Brisman MD
Chocolate City Burlesque
Advanced Electric Scooters
W Tougei
Aljouf Now
Arrister
Bruce Holmes Construction
Burlington Bridal
Chocolate Creative Design
Culture of Life Store
Elizabeth Cryan Photography
Fun Fresh Ideas
Kotoyuujin
Lyudia
Nakakirei
Peer AGS
Maimun88
Sinsei PV
Think Tank West
Wimax Gogo
Asperger Way
Book Rack WA
Hume Coover Studio
Pico Compressor
PPTAA
Artina Films
Bash Booths
Bicher Cancer Institute
Brickyard Theatre
Coffee with Jeff
Essential Libertarianism
Find the Light Within
Font Plus Tips
GB Product News
Homer's Auto Services
Luxellence Center
Matome Hannou
Nepal Smoking Pipe
OEM Korea Brand
Shoe Saxo
The Stock Market Blueprint
Untapped Inc.
Andres Carizza
Ashland Aerial
Brown About Town
REH Shows
Shotover Country
Toni Halonen
Trainers P
gayahidupsehat.org
Bulging Disc Fixed
Cadogan Cosmetics
D Chavannes
Kilo Pages
Loring Military Heritage Center
Modular Homes MA Planning Center
The Wheel Herb Farm
Bxtches Be Blogging
CCTV Installers London
HiFi Noise
iGo Inter
Payroll Services UK
Shop Moraga First
The Highline Restaurant
Visval Bags
BR Fittings
HD Fit Pro
Regalos a Peru
Shot Felt Round the World
BG Receptite
Photography Travel
Desperate Endeavors Movie
JetEx Charter
Amenity Papa
iSoftol
Pagar Besi
Oakfield BnB
Delight Worthy
Mantis Plant Protetion
Unryuuji
Wamsted On Energy
Doctor McAwesome
WHDMS
Jual Aged Domain
Gimena Macri
Rancho de Caldera
Vietnam Tours Lotus
A Little Bit OCD
Kingston Single Malt Society
CEI Spokane
Research Enterprises
Escape the Stall
Nippon Touhan Club
Pro Point Dies
Basement Metal
Boeren Boedel
BW Mariposa Inn
Growing Up Online
Heartland Baptist Church
Kandee Chihuahuas
Lubov Kirillovka
Lucky Dog Grooming and Boutique
Nihon Megane
Osiris The Series
Polychromatic Game
Soccer Planet JWT
Suzy Wimbourne Photography
The Hollywood Garage
Travel Alchemy